AIOps @ SEB
In SEB’s AIOps-themed blogposts we will dig deeper into what AIOps at SEB is, some of the prerequisites, what it means to work with AIOps and what the future holds.
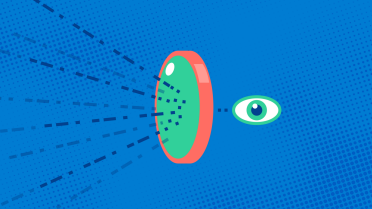
Hi again! In the last blog post from AIOps we explained why we are focusing on AIOps at SEB. Now we’d like to dig into what we have worked on previously.
Our first use case was focused on creating temperature anomaly detection in our data centres to enable earlier insights of temperature changes that might impact our hardware and the services we provide. Together with other teams at the bank, we extracted temperature data and then, through in-house development, we created solutions for pre-processing of data, anomaly-detection implementation using statistical models, and post processing to make sure that the correct teams get notified in case of anomalies.
Using actual data instead of hunches as the basis for decisions will in theory lead to better insights, better decisions and thriving businesses. But what happens if the data you are using does not have the quality you expect? Let’s say you’re in a meeting with 500 participants and you ask them what their favourite ice cream is. You might get answers showing 40% chocolate, 40% vanilla and 10% more unique flavours, and the last 10% being a mix of animal names, numbers or a lack of answers because the participants were emailing at the same time and did not actually catch what the question was. If you apply machine learning and automations using this data, you might end up delivering animals to an ice cream shop.
For machine-generated data we do not encounter this exact issue. But there are still issues that can occur: data not being sent at regular intervals, a lack of data points, or man-made outliers – maybe someone accidentally blocked temperature sensors? This is where data investigation and pre-processing are crucial, and we spent a lot of time making sure data quality was good and creating rules for outliers before we started with the anomaly detection part.
For this use case we investigated several ML/statistical models to see which one was the best fit. Some were excluded because they consume unnecessary amounts of resources and some were excluded because they did not give enough accuracy. It’s important to keep in mind to use the best models for your use case - having accuracy and resource use in mind and not just choosing the ones that sound cool. In the end, we chose a statistical model that was very efficient and a great fit for this use case.
During the post- processing phase we created events on certain anomaly thresholds and connected these to the event management system in the bank. As a result, this use case provided us with earlier insights to temperature changes in our data centres, and as a bonus we enabled identification and remediation of hot spots. This was a great case to test our wings, but we have since moved on to implementing intelligent observability for one of our largest Kubernetes environments, containing thousands of containers and pods. This is something we will talk more about in our next blog post – see you then!
In SEB’s AIOps-themed blogposts we will dig deeper into what AIOps at SEB is, some of the prerequisites, what it means to work with AIOps and what the future holds.
How do you set up and maintain an environment where you adhere to the regulations while at the same time ensuring that you have empowered employees?
I think some still remember when non-functional requirements (NFR) were activities in a project plan and thus could be prioritized accordingly.